This Work Package deals with the analysis of plant data and neutron current and flux modelling, through advanced signal processing and machine learning techniques. In particular, signal processing methods include Fourier and Hilbert transformations, multi-resolution wavelet analysis, while machine learning focuses on deep learning and deep neural networks (DNNs). Specific targets include the detection of abnormal fluctuations & their classification, handling of scarcity of in-core instrumentation, learning in selected reactor(s) and transferring it to others adaptation of the developed techniques towards their application to real data.
- 2 types of perturbations
- many locations and sensors
- large amounts of data
- 1 type of perturbation
- 4 locations and tenths of sensors.
CORTEX work presented at the World Congress in Computational Intelligence
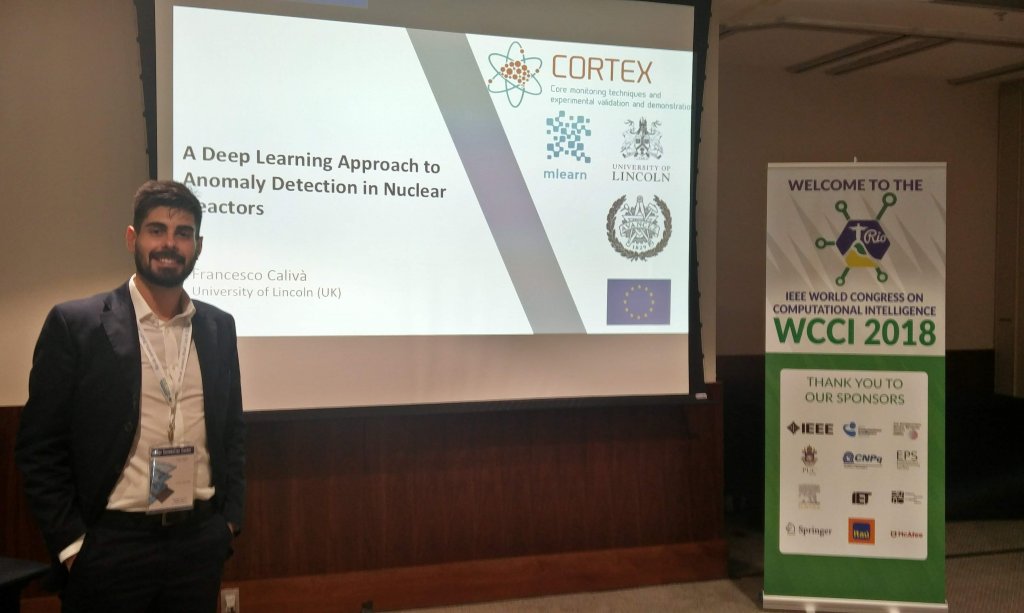